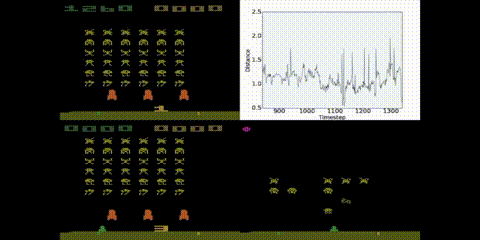
Scalable methods for computing state similarity in deterministic MDPs
This post describes my paper Scalable methods for computing state similarity in deterministic MDPs, published at AAAI 2020. The code is available here.
Motivation
We consider distance metrics between states in an MDP. Take the following MDP, where the goal is to reach the green cells:
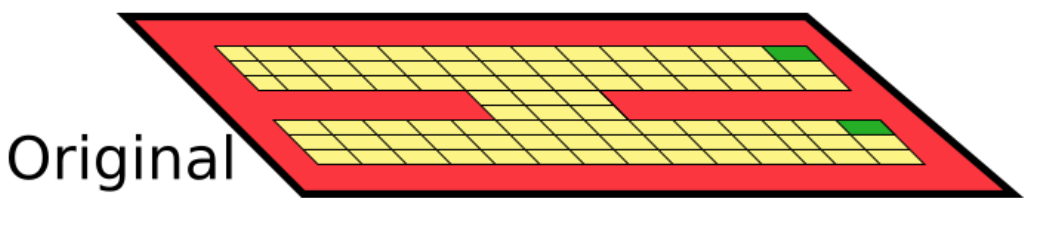
Physical distance betweent states?
Physical distance often fails to capture the similarity properties we’d like:
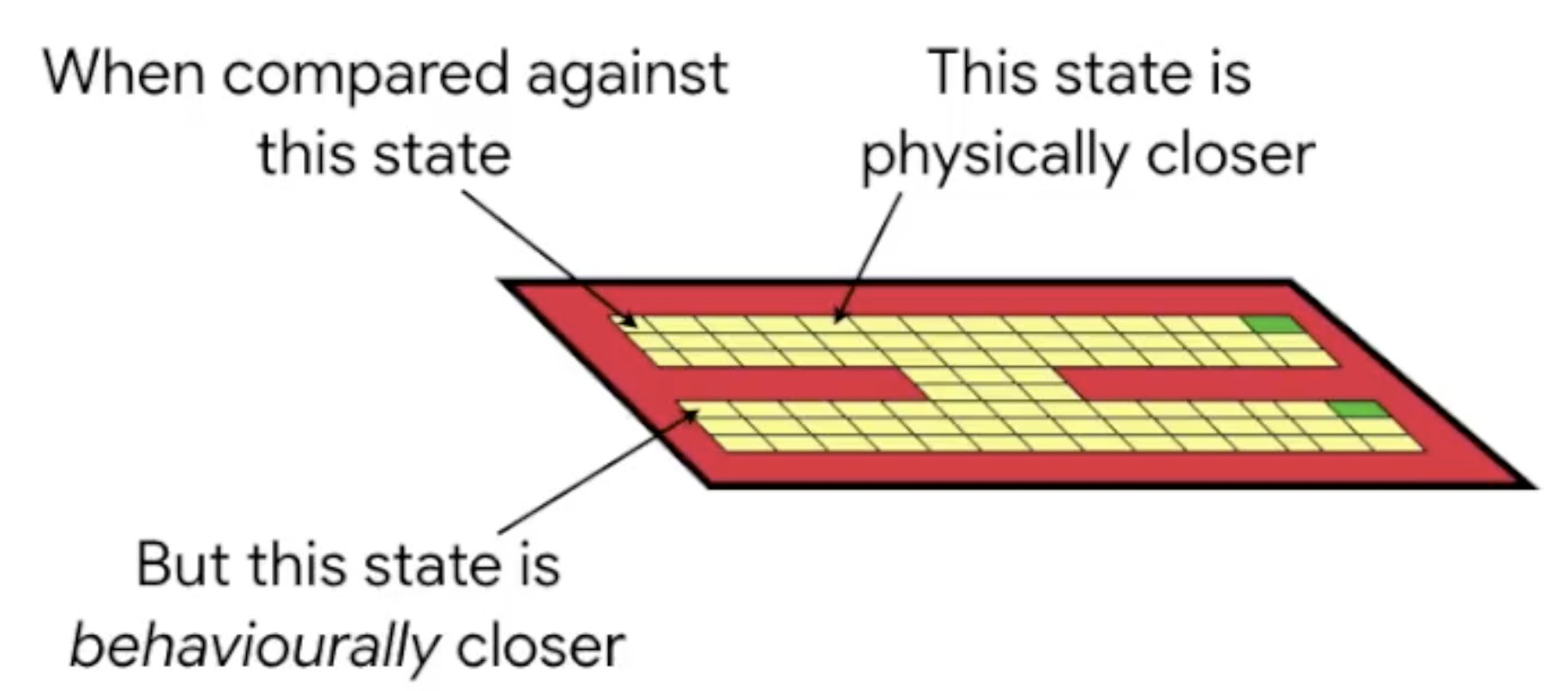
State abstractions
Now imagine we add an exact copy of these states to the MDP (think of it as an additional “floor”):
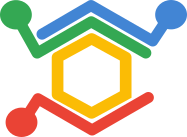
Dopamine: A framework for flexible value-based reinforcement learning research
Dopamine is a framework for flexible, value-based, reinforcement learning research. It was originally written in TensorFlow, but now all agents have been implemented in JAX.
You can read more about it in our github page and in our white paper.
We have a website where you can easily compare the performance of all the Dopamine agents, which I find really useful:
We also provide a set of Colaboratory notebooks that really help understand the framework: