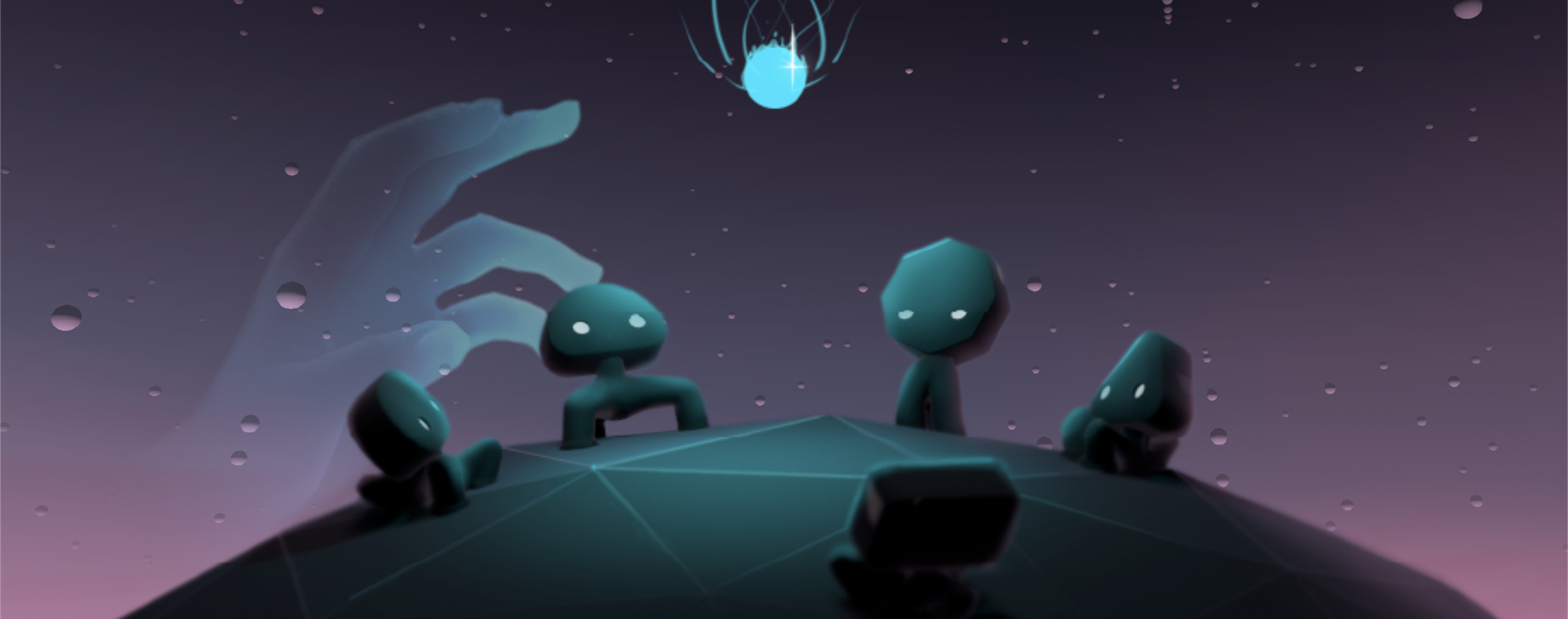
Agence: a dynamic film exploring multi-agent systems and human agency
Agence is a dynamic and interactive film authored by three parties: 1) the director, who establishes the narrative structure and environment, 2) intelligent agents, using reinforcement learning or scripted (hierarchical state machines) AI, and 3) the viewer, who can interact with the system to affect the simulation. We trained RL agents in a multi-agent fashion to control some (or all, based on user choice) of the agents in the film. You can download the game at the Agence website.
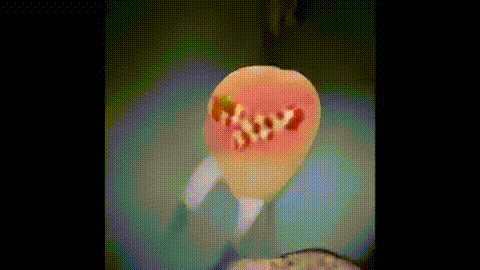
GANterpretations
GANterpretations is an idea I published in this paper, which was accepted to the 4th Workshop on Machine Learning for Creativity and Design at NeurIPS 2020. The code is available here.
At a high level what it does is use the spectrogram of a piece of audio (from a video, for example) to “draw” a path in the latent space of a BigGAN.
The following video walks through the process:
GANs
GANs are generative models trained to reproduce images from a given dataset. The way GANs work is they are trained to learn a latent space $ Z\in\mathbb{R}^d $, where each point $ z\in Z $ generates a unique image $ G(z) $, where $ G $ is the generator of the GAN. When trained properly, these latent spaces are learned in a structured manner, where nearby points generate similar images.
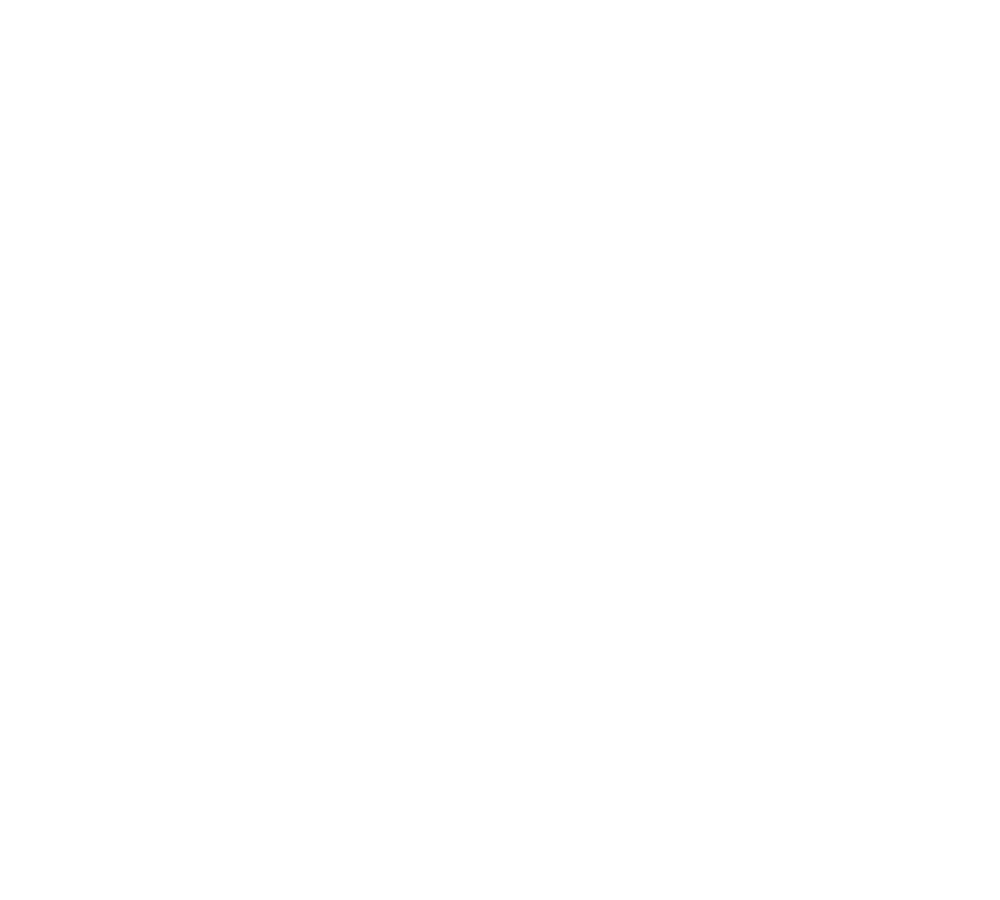
Rigging the Lottery: Making All Tickets Winners
Rigging the Lottery: Making All Tickets Winners is a paper published at ICML 2020 with Utku Evci, Trevor Gale, Jacob Menick, and Erich Elsen, where we introduce an algorithm for training sparse neural networks that uses a fixed parameter count and computational cost throughout training, without sacrificing accuracy relative to existing dense-to-sparse training methods.
You can read more about it in the paper and in our blog post.
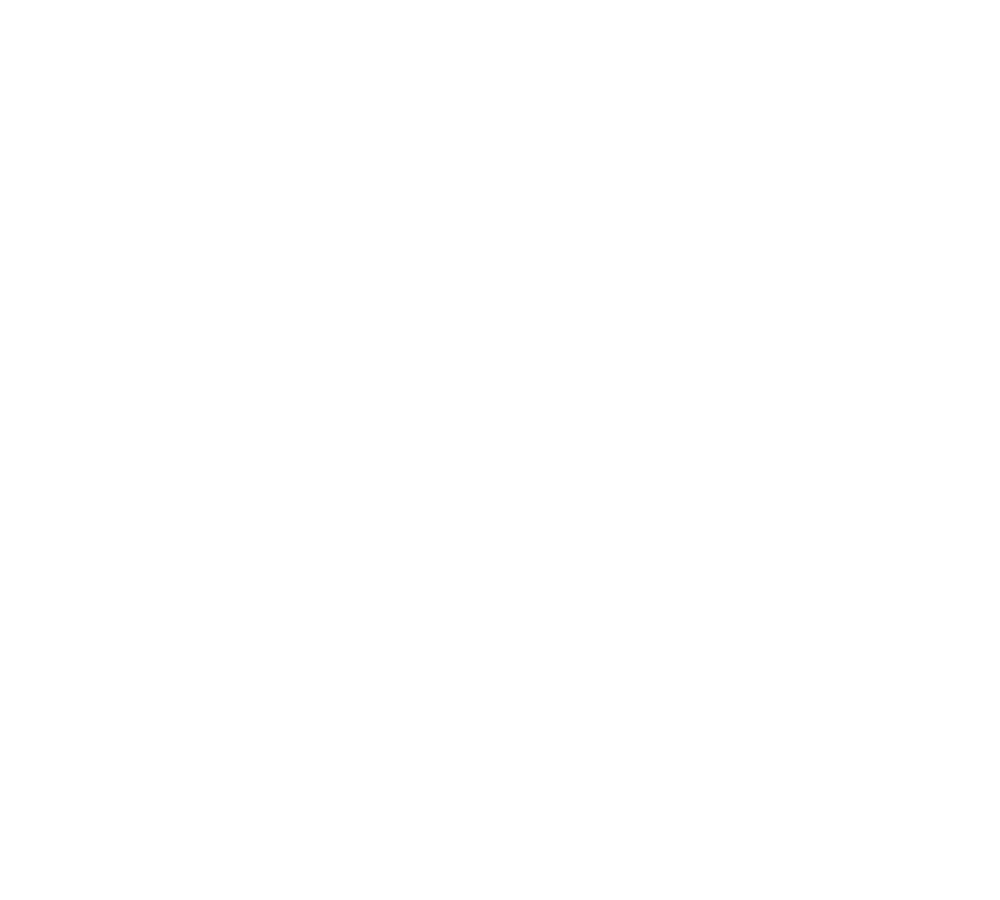
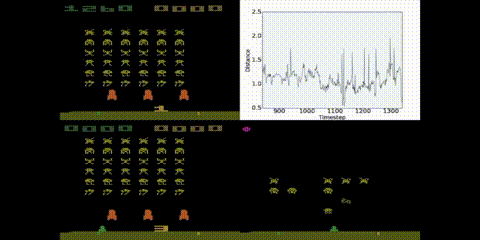
Scalable methods for computing state similarity in deterministic MDPs
This post describes my paper Scalable methods for computing state similarity in deterministic MDPs, published at AAAI 2020. The code is available here.
Motivation
We consider distance metrics between states in an MDP. Take the following MDP, where the goal is to reach the green cells:
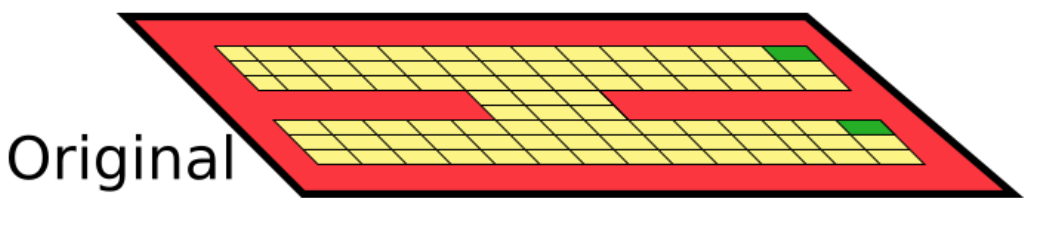
Physical distance betweent states?
Physical distance often fails to capture the similarity properties we’d like:
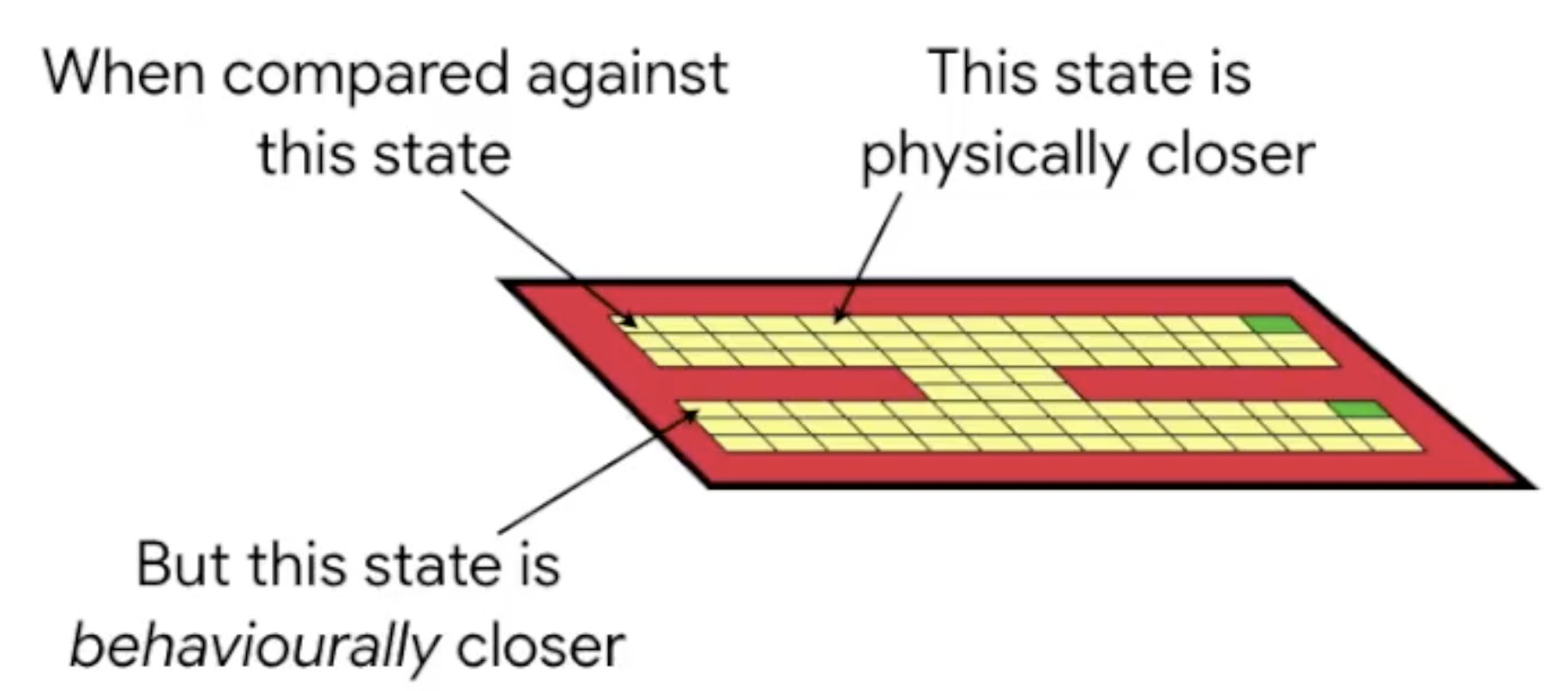
State abstractions
Now imagine we add an exact copy of these states to the MDP (think of it as an additional “floor”):
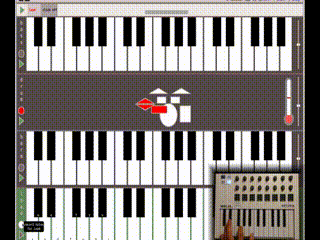
ML-Jam: Performing Structured Improvisations with Pre-trained Models
This paper, published in the International Conference on Computational Creativity, 2019, explores using pre-trained musical generative models in a collaborative setting for improvisation.
You can read more details about it in this blog post.
You can also play with it in this web app!
If you want to play with the code, it is here.
Demos
Demo video playing with the web app:
Demo video jamming over Herbie Hancock’s Chameleon:
Demo video over free improvisation:
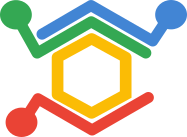
Dopamine: A framework for flexible value-based reinforcement learning research
Dopamine is a framework for flexible, value-based, reinforcement learning research. It was originally written in TensorFlow, but now all agents have been implemented in JAX.
You can read more about it in our github page and in our white paper.
We have a website where you can easily compare the performance of all the Dopamine agents, which I find really useful:
We also provide a set of Colaboratory notebooks that really help understand the framework: